Case Study
Orbital: Decentralised Deep Learning sets New State of the Art benchmark in asset failure prediction by 52%
12 Jun 2024
Goal
Demonstrate that a decentralised deep learning approach for asset failure prediction can be achieved while maintaining data privacy, security, and reduced operational costs.
Develop and evaluate this approach in a digital twin environment for pipeline failure prediction up to 96 hours before onset.
Benchmark and compare this approach to current machine learning methods deployed via Cloud in the industry on the following metrics: Model Accuracy, False Positive Prediction and Prediction performance
Challenges
The following are the Data science challenges our approach overcame:
~2.5 exabytes of data generated routinely, and yet not fully utilised by models
Optimization methods assume steady state operations even in dynamic states (refinery processes)
Models require step-test periodic calibration which takes months
Model flexibility is limited, as they only operate in restricted regions
Results
Overall Accuracy: The Orbital deep learning model shows the highest performance with an accuracy of 95.12% predicting pipeline failure, 4 days before advance.
Daily Prediction Accuracy: 52% higher accuracy at each day of prediction.
False Positives: 67% fewer false-positive predictions.
Prediction Speed: 427% earlier predictions compared to other models.
Background
In recent years there has been a surge in predictive maintenance activity in the Oil and Gas Industry - advanced machine learning models allow for better prediction to mitigate potential issues before they escalate.
Recent advancements in IoT data and 5G networks provide significant opportunities for improvement, allowing for more comprehensive data collection and faster transmission. However, these advancements also introduce challenges in scaling deep learning models due to the volume and diversity of data. As a result, Industry Standard models only utilise a fraction of the available data whilst hindered by expensive infrastructure and data security issues.
Orbital’s Development and Implementation
We developed a smart AI infrastructure that enables training, and deployment of deep learning solutions for predictive modelling of refinery operations. Our deep learning models are deployed on an edge computer which sits between, where the refinery stores its data (historian) and where engineers view it (SCADA).
Our models read real-time data from the refinery's storage, process it on the device and send results back to the display system. Our software on this device allows data scientists to train and run any deep learning models right at the refinery, without needing to send data elsewhere.
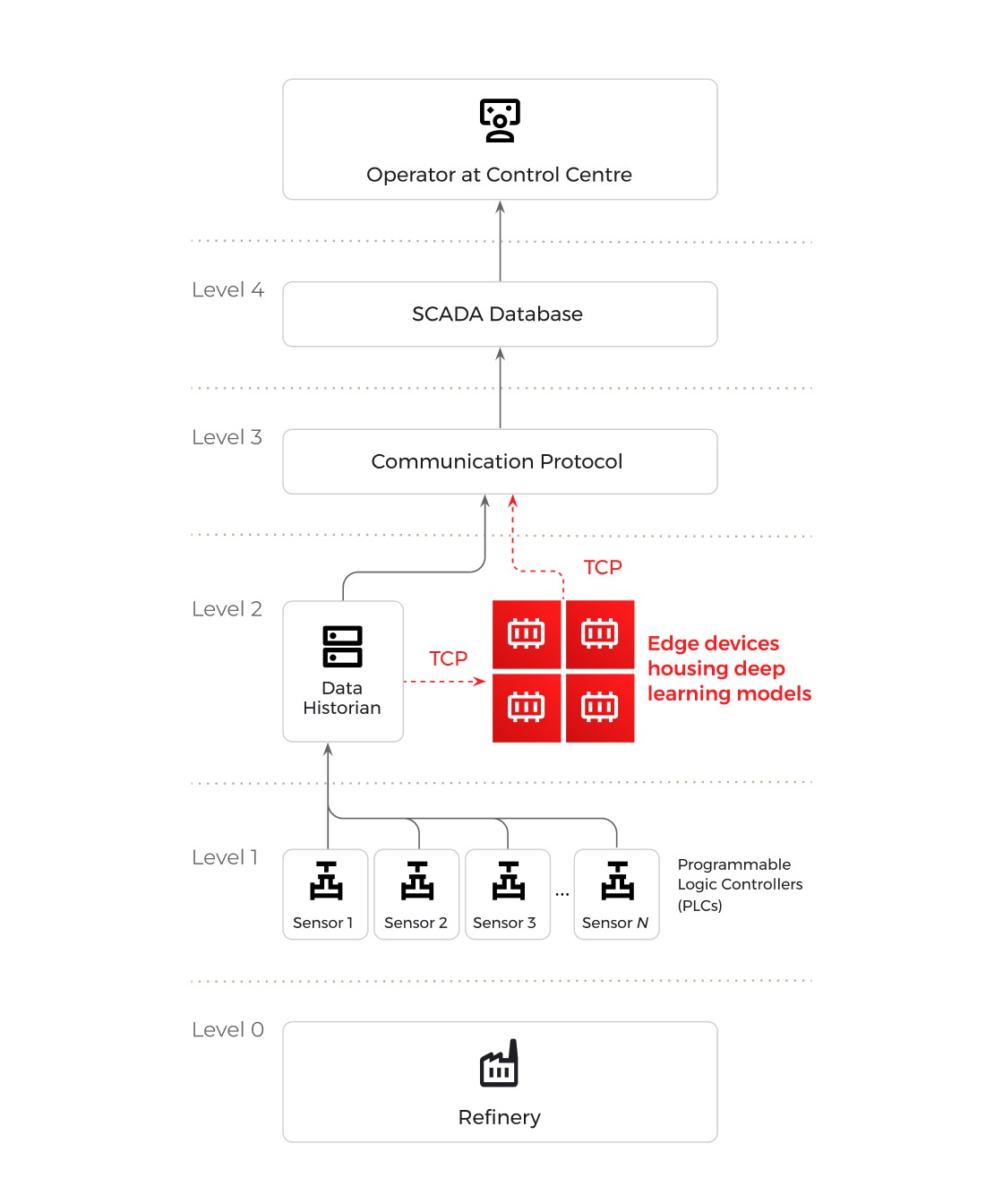
This setup allowed us to train a predictive deep learning model immediately on our edge devices and deploy it in a digital twin for evaluation whilst keeping the data secure and integrating smoothly with the twin’s backend systems. In our study, we deploy a transformer model for:
Create an accurate predictive model
Forecast pipeline failures within an 8 time-interval windows (12 to 96 hoursEnsure Efficient and Effective Model Training
Tailor specific parameters (model weights, weight decay, warm-up epochs, momentum parameters, and learning rates) for both pre-training and prediction fine-tuning phases.Build a Noise-Resilient Model
Capture both short-term and long-term temporal dynamics using masked-data modelling and random stride sampling.
Orbital benchmarked against industry standard machine learning and deep learning models
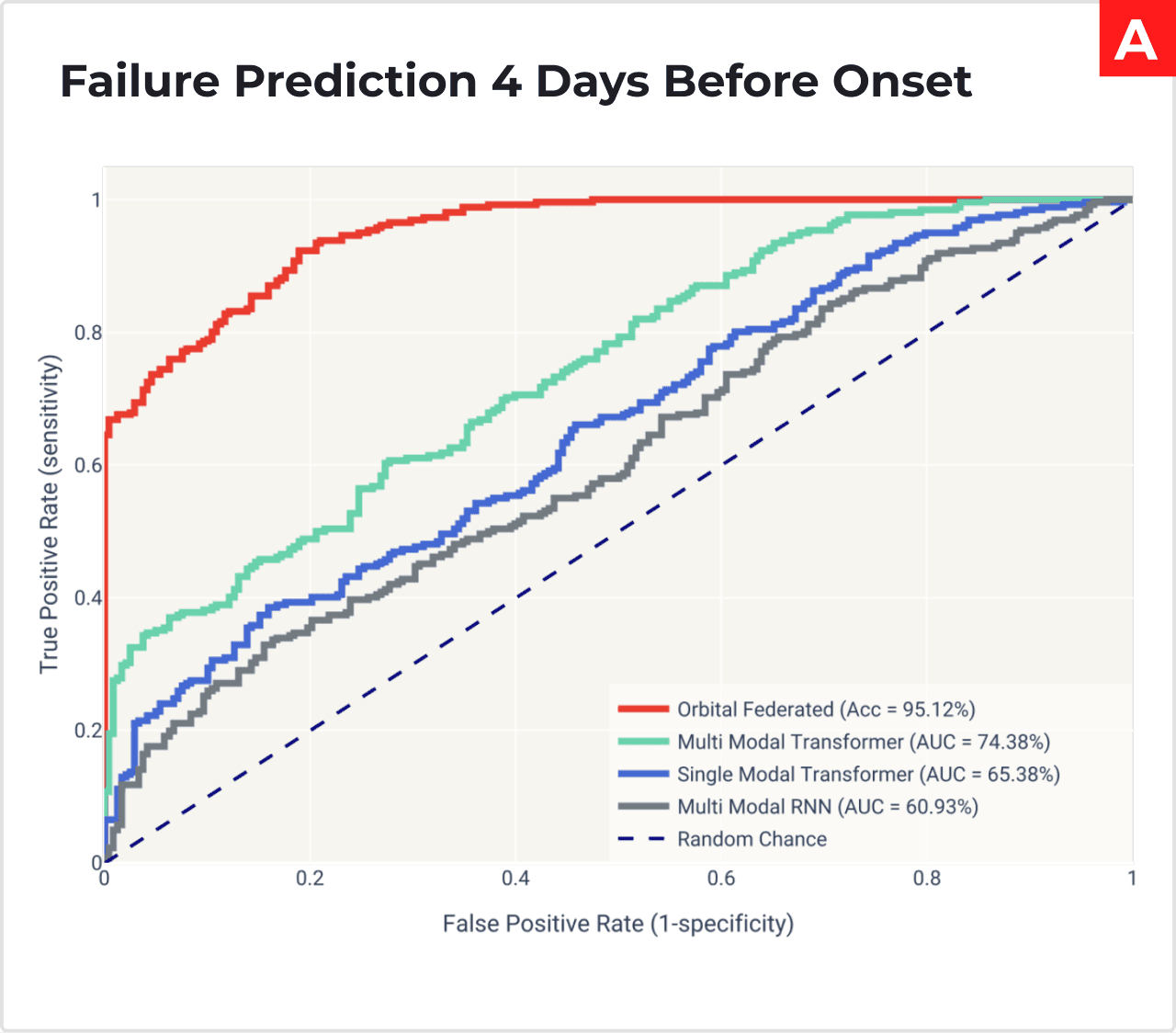
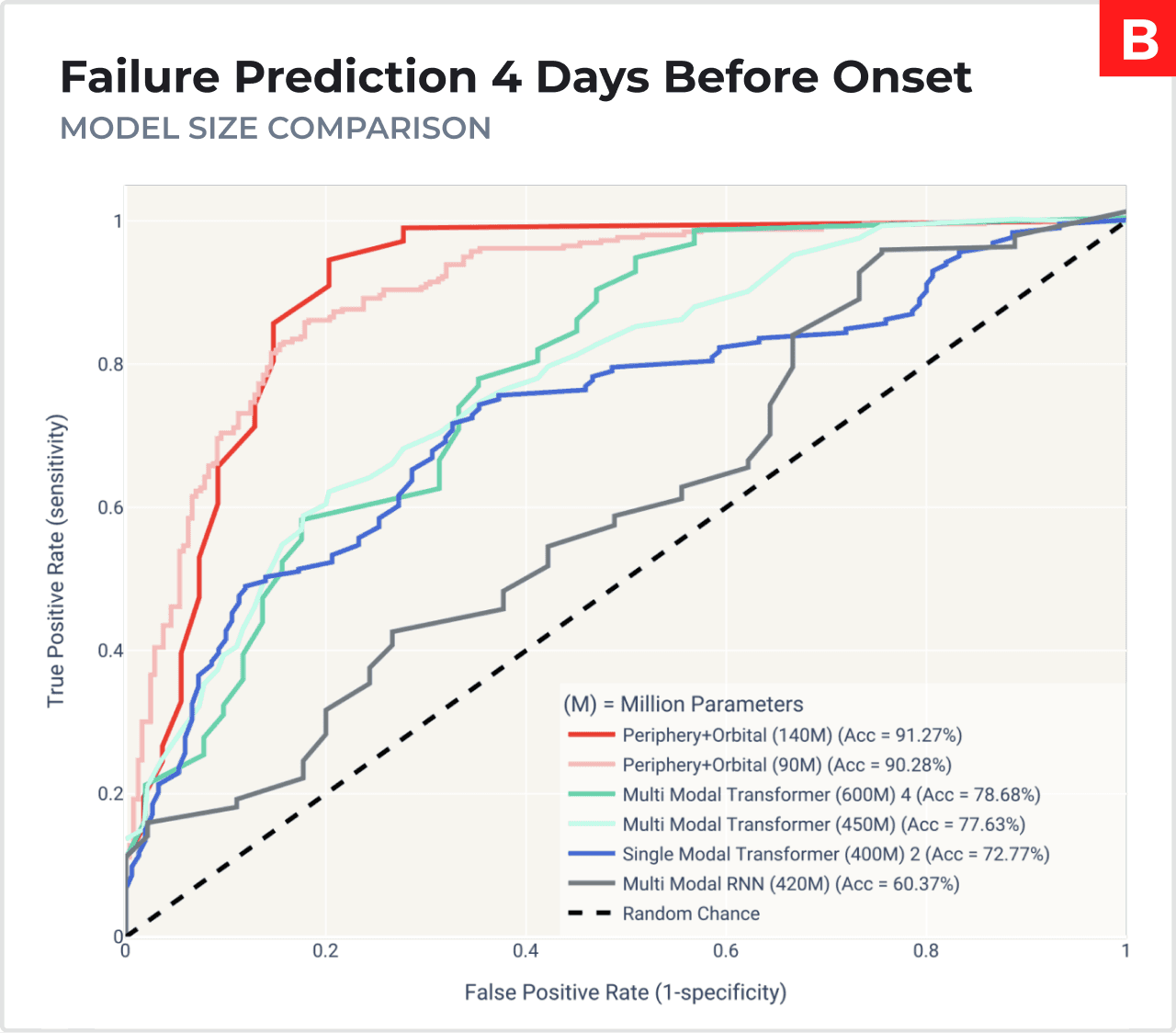
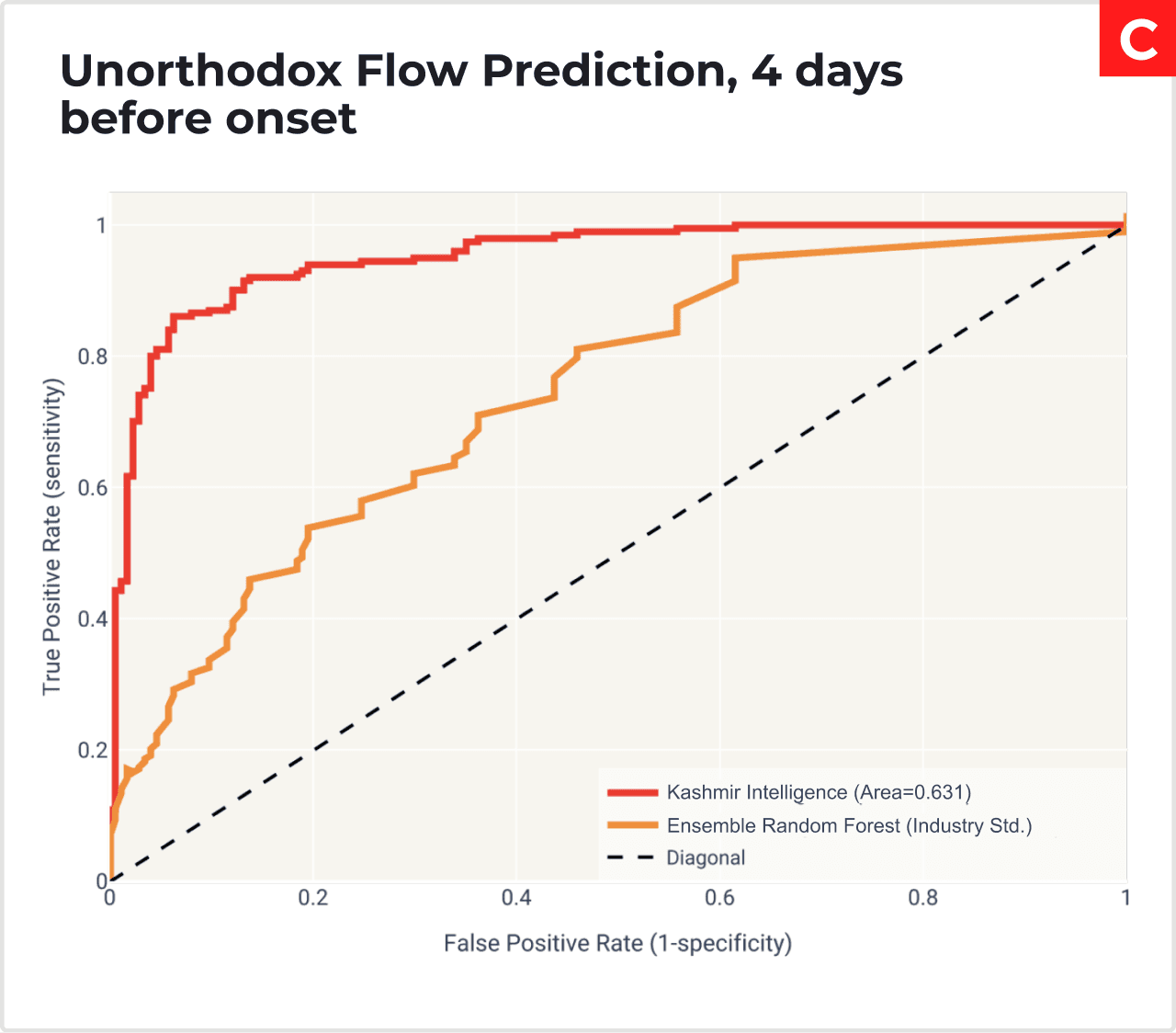
The graphs A-C showcase the Receiver Operating Characteristic (ROC) curves of Orbital being compared to other
State-of-the-art methods. (A) displays Orbital compared against other deep learning models. (B) Shows Orbital compared with different sizes of deep learning models. In both A and B, competing models were trained in centralised fashion via supervised learning. In (C) Orbital is compared with Industry standard model: Ensemble of Random Forests. Area of the curves is the accuracy of the model
Conclusions
Orbital has proven to be a superior method for building predictive models. This advancement is crucial for the oil and gas industry, as it addresses many of the current challenges, such as deploying dynamic state-of-the-art models, data under utilisation, high costs, and security concerns.
By overcoming these obstacles, Orbital enables more efficient and effective predictive maintenance, thereby enhancing overall operational efficiency.
Data Utilisation: Orbital uses 100% of real-time data resulting in higher performing models.
Model Performance: Dynamic deep learning model that predicts failures 8 times earlier than current rates.
Speed to Install: Models can be built and optimised within weeks, significantly reducing deployment time.
Cost Effective: While current deep learning methods rely on cloud infrastructure, decentralised models can be implemented on location for a 75% cost-reduction versus cloud data systems.
Data Security: Unlike cloud, on-prem training ensures that data never leaves existing infrastructure resulting in reduced security risks
STAY UP TO DATE